Task 1
Design requirements of the integrated system “Monitraffic”
Task 1 assessed the current practice on traffic analysis and monitoring and identified the needs and requirements of the tools to be developed in the scope of the project. Different needs and options of a data-based maritime traffic analysis platform for ship trajectory data provided by the Automatic Identification System (AIS) have been discussed in an initial project workshop on “Monitoring and Surveillance of Maritime Traffic off the continental coast of Portugal” held on 25th November 2020 at IST.
The workshop gathered several relevant stakeholders from the local maritime community, such as, representatives of the VTS control centre and EMSA, as well as ship operators and the research team. The needs in terms of tools and information to support their activities were identified. The benefits of the developed tools in the project were demonstrated.
The introduction of the Automatic Identification System (AIS) by IMO in 2004 [1] has provided an immense level of detail over maritime operations at sea. SOLAS regulation v/19 establishes the requirement for deployment of AIS transponder on board ships.
Coastal stations, such as Vessel Traffic Service (VTS) centres or Rescue Coordination Centres (RCC), are among those who most benefit from AIS. This system expands VTS’s control range and functions, enhancing identification, tracking and vessel monitoring capabilities [2]. AIS data received from ship stations can be recorded and subsequently used to study maritime traffic, making it an exceptionally valuable tool. AIS is also used for Search and Rescue (SAR), namely by Rescue Coordination Centres (RCC), SAR vessels and aircrafts, or on AIS Search And Rescue Transponders (AIS-SART) and AIS Man Overboard (MOB) devices. Aids to Navigation (AtoN) can also be equipped with AIS, which improves and enhances services provided to mariners [3].
Since AIS messages are transmitted over VHF, its range is limited in practice by the curvature of the earth and by the power of the transmitter. However, the “line-of sight” limitation for earth stations may be overcome by the use of Satellite based AIS (Sat-AIS) [4]. In the case of Sat-AIS, the average interval between available reports is between 4 and 5 hours, much longer than Terrestrial based AIS (T-AIS).
There have been some security concerns with AIS, as the system is vulnerable to security threats, such as spoofing, hijacking and availability disruption [5]. Possible spoofing (faking the message source) attacks to AIS include ship spoofing, AtoN spoofing, SAR spoofing, CPA spoofing, distress beacon spoofing or faking weather forecasts. Hijacking attacks involve tampering with
information from existing AIS stations. Availability disruption attacks prevent normal communication between AIS stations and may include slot starvation, frequency hopping and timing attacks.
AIS information is available on the internet in some popular websites like MarineTraffic.com, AIS Hub, Vessel Finder, and others. IMO’s Maritime Safety Committee (MSC) considers the publication
of AIS data on the world-wide web or elsewhere detrimental to the safety and security of ships and port facilities, urging Member Governments to discourage those who make such data available for publication and condemning those who publish the data [6].
While the public availability of AIS information has raised security concerns, researchers have been taking advantage of this source of data to study subjects as diverse as traffic, logistics and transport economy and efficiency, collisions, emissions, oil spills, noise, interaction with whales, fishing, ice and monitoring.
AIS messages are transmitted in an encoded format and must be decoded in order to be interpreted [7]. The AIS data used in the scope of this work was recorded by Centro de Controlo de Tráfego Marítimo do Continente (CCTMC), the Portuguese coastal VTS centre.
The integrity of all AIS messages can be verified using a checksum, which is found at the end of each message. However, it is possible to find messages with correct checksums, but wrong bit length for the specified message type [8]. In addition, a number of other errors may be found in AIS data, resulting from poor human input, incorrect installation or faulty sensors.
Data inconsistencies (position inconsistencies in particular) are a major setback when using AIS, and they should be dealt with before the data can be used. Regarding the data used for this research work, the following problems were detected:
- Simultaneous position reports (message types 1, 2 and 3) transmitted by the same MMSI containing different position information (latitude and longitude);
- Position reports in which the position information was inconsistent with the previous position report from the same ship, given the course and speed at which the ship was proceeding. In some cases this could be visualized as a “back and forth“ type of behaviour.
To address data validity and consistency issues, a pre-processing procedure was developed and implemented.
Maritime traffic assessment based on AIS historical data provides a capacity to model the ship’s navigation route and consequently the ship’s regular movement pattern [9]. The vessel that does not follow the common pattern turns automatically as suspicious, because the irregular motion is less predictable, hence, presents higher collision risk. This knowledge makes real time anomaly detection valuable tool for vessels and port authorities, as it identifies potential security and navigation safety hazards. To model maritime routes by extraction of necessary information from AIS data, three types of methods are available:
- Grid-based, e.g., Vettor and Guedes Soares (2014) [link], Bomberger et al. (2006) [link];
- Vector-based, e.g., Pallotta et al. (2013) [link], Kaluza et al. (2010) [link], Coscia et al. (2018) [link];
- Statistic-based methods, e.g. Silveira et al. (2013) [link], Wu et al. (2016) [link], Kang et al. (2018) [link].
To develop ship’s regular movement pattern, the maritime traffic normalcy is extracted from AIS historical motion data by statistical methods [10]:
- Parametric, e.g., Gaussian mixture models;
- Nonparametric, e.g., Kernel density estimation;
- Clustering, e.g., density based spatial clustering.
If the ship is located outside the normalcy, the corresponding ship position or the entire trajectory can be identified as anomalous by point-based anomaly detection methods and trajectory-based anomaly detection methods, respectively.
The term anomaly is used in many domain areas with different meanings. Anomaly is typically associated with terms such as abnormal, unusual, irregular or illegal. Generally, an anomaly always represents a deviation from normality (i.e., usual, regular, typical). In the maritime domain, the more common marked anomalies are [10]:
- Positional – vessels travelling outside the standard route in unusual areas;
- Contextual – vessel’s behaviour is directly correlated to the context (e.g., correlation of anomalies with time-related concepts such as seasons, days of the week, workdays vs holidays);
- Kinematic – vessels showing a wrong direction or unusual course over ground such as sailing in the opposite direction on an established route, instantaneous stops and turns;
- Complex – vessels engaged in illegal activities;
- Data-related anomalies – incomplete trajectory data.
Based on the data processing strategy, the maritime anomaly detection methods can be split into three groups [10]:
- Data-driven approaches. A maritime traffic normalcy model is constructed based on historical ship trajectories. The approach assumes that most of the historical trajectories reflect the “normal” behaviour of ships;
- Signature-based (or rule-based) approaches look for predefined patterns, positions, or dynamic characteristics on the ship trajectories (e.g., U turns, low speed, course to coast) and do not explicitly rely on historical maritime traffic information. This approach uses simple rules to identify anomalies (e.g., IF THEN ), and is adequate for real-time applications in monitoring systems;
- Hybrid approaches combine data and knowledge-driven approaches in a semi-supervised way.
Various commercial applications were developed for maritime anomaly detection:
In 2014, EMSA launched Integrated Maritime Services (IMS) platform to ensure the performance, availability and reliability of all the maritime information systems it hosts. IMS combines maritime data from different reporting/tracking vessel systems, Earth Observation satellite remote sensing products, Automated Behaviour Monitoring (ABM) alerting tools, Enhanced Search & Rescue functionality and a wide range of met-ocean related information.
Automated Behaviour Monitoring is the IMS tool, rule-based system that analyses ship positions for the automatic detection of abnormal and/or specific vessel behaviour [11]. ABM analyses ship position reports using data from available tracking systems and has over 20 different algorithms for different abnormal behaviour patterns.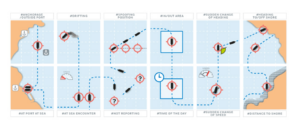
In order to improve ABM’s efficiency, EMSA in close cooperation with ABM working group elaborated the plan of priorities for the data analytics. The outcome consists of data sets, derived products, and their possible application assessments. It is found that some of the high priorities for ABM development are in line with the MoniTraffic plan of work and objectives, such as the implementation of the ABM based on the historical data analysis (i.e. alerting when specific behaviours are detected on the historical track of the vessel of interest)
ABM features to be developed as of 2020 [11]:- Anomaly detection based on the historical data analysis;
- Detection of destination change and navigational status using AIS transmitted data;
- Prediction of the ship movement: now casting and future casting;
- Detection of the navigational status – Not Under Command;
- Combination and inclusion of multiple vessel attributes and elements, such as: LOA, year of built, GT, danger goods (HAZMAT), flag change, owner information, fishing vessels identifiers;
- Combination of ABM scenarios by user;
- Detection of deviation from the most usual route;
- Automatic assignment of the vessel risk based on static and dynamic data sets;